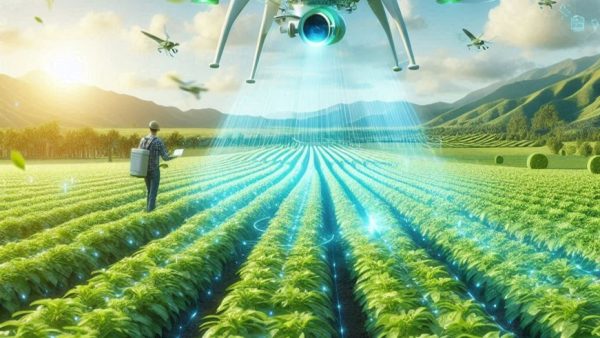
Impact of AI in Agricultural Industry And Challenges
AI is playing an increasingly significant role in the agriculture industry worldwide, transforming traditional farming practices into more efficient, productive, and sustainable ones.
The future of AI in agriculture promises to enhance productivity, sustainability, and resilience in farming, helping to address global challenges such as food security, climate change, and environmental sustainability. As technology continues to evolve, its integration into agriculture will bring about transformative changes, making farming smarter, more efficient, and more sustainable than ever before.
Ways AI impacting current agriculture practices –
Precision farming
- Soil and crop monitoring: AI uses sensors and IoT devices to collect data on soil moisture, nutrient levels, and crop health. This data helps in precise application of water, fertilizers, and pesticides.
- Variable rate technology (VRT): AI algorithms determine the optimal number of inputs like seeds, fertilizers, and chemicals to be applied at specific locations in the field, reducing waste and costs.
Predictive analytics
- Yield prediction: AI models analyze weather patterns, soil conditions, and crop health data to predict yield outcomes, allowing farmers to plan better.
- Disease and pest forecasting: AI systems can predict the likelihood of disease outbreaks or pest infestations, enabling timely preventive measures.
Automation and robotics
- Autonomous equipment: AI-powered tractors and machinery can perform planting, weeding, and harvesting tasks autonomously, improving efficiency and reducing labor dependency.
- Harvesting robots: Robots equipped with AI can identify and harvest ripe fruits and vegetables, minimizing damage and increasing productivity.
Drones and satellite imaging
- Aerial surveillance: Drones equipped with AI analyze aerial images to assess crop health, identify stressed areas, and monitor irrigation levels.
- Remote sensing: Satellites provide large-scale images and data, which AI analyzes to monitor crop growth, soil conditions, and environmental changes.
Supply Chain Optimization
- Demand forecasting: AI predicts market demand for various crops, helping farmers decide what to plant and when to harvest.
- Planning: AI optimizes the supply chain by managing inventory, reducing waste, and ensuring timely delivery of fresh produce.
Livestock management
- Health monitoring: AI systems monitor livestock health through wearable devices and cameras, detecting signs of illness, stress, or abnormal behavior early.
- Feeding optimization: AI analyzes data on feed intake and growth rates to optimize feeding schedules and compositions.
Climate change adaptation
- Weather prediction: AI models predict short-term and long-term weather patterns, helping farmers make informed decisions about planting and harvesting.
- Resilient crop varieties: AI research aids in developing crop varieties that are more resistant to drought, pests, and diseases.
Smart irrigation systems
- Efficient water use: AI-powered systems use real-time data to determine the precise amount of water needed for crops, reducing water waste.
- Automated irrigation: AI controls irrigation systems to water crops at optimal times, ensuring better growth and yield.
Market forecasting
- Price prediction: AI analyzes market trends to forecast prices of agricultural products, helping farmers decide the best times to sell their produce.
- Consumer preference analysis: AI identifies consumer preferences and trends, guiding farmers on what crops to grow for better profitability.
Resource management
- Optimizing inputs: AI helps in the efficient use of resources like seeds, fertilizers, and pesticides by determining the exact quantities needed.
- Environmental impact reduction: AI promotes sustainable farming practices by minimizing the overuse of chemicals and conserving natural resources.
Several leading agricultural companies are leveraging AI technology to innovate and enhance their farming practices are – John Deere, Bayer, Syngenta, Corteva Agriscience, Trimble Agriculture etc. In India – Salam Kisan, DeHaat, Ninjacart, Cropin Technology, AgNext are examples where AI is using AI to help farmers. These companies are at the forefront of integrating AI into agriculture, driving innovation, and helping farmers meet the challenges of modern farming through advanced technology and data-driven solutions.
Challenges faced by AI supported Ag Industry:
1. High Initial costs of technology investment – this is a big challenge mainly small-scale farmers face.
2. Data management and quality which requires a vast amount of accurate and comprehensive data.
3. Technical complexity of understanding AI technologies and knowledge by farmers including maintenance and support
4. Infrastructure Limitations like connectivity issues for AI Apps through network and power supply
5. Regulatory and ethical concerns – data protection and privacy concerns by farmers. Data should be ethical.
6. Regulatory compliance: Navigating drones and autonomous machinery through AI can be complex.
6. Environmental and social Impact – it is important to ensure farmers that these technologies promote sustainable practices.
7. Automation of the farm work due to AI application could cause Job displacement and can lead to social and economic concerns.
8. Reliability and accuracy of AI based models – if there is incorrect recommendation or predications it could lead to heavy losses. Ensuring farmers with practical knowledge under varying conditions that AI technology works is essential.
9. Economic barriers – small scale farmers might not be able to afford the technology. Another major challenge is funding and investment.
10. Cultural and behavioral resistance – farmers who rely on traditional practices might be hesitant to adopt innovative technologies. Building trust with transparency approaches is critical to introduce AI technology in the field.
Addressing these challenges requires a concerted effort from technology developers, policymakers, educational institutions, and the farming community to create a supportive ecosystem for AI in agriculture.
References:
- https://www.nifa.usda.gov/artificial-intelligence
- https://www.forbes.com/
- https://www.mckinsey.com/industries/agriculture
- https://www.basic.ai/blog-post/7-applications-of-ai-in-agriculture
- https://krishijagran.com/
Image credit: Generated with AI ∙ July 31, 2024, at 7:57 PM
Author: Sumana Rao | Posted on: August 1, 2024
« Farming In India Offers Profitable Opportunities Reasons For Global Popularity Of Basmati Rice »
Write a comment